Our websites use cookies. By continuing, we assume your permission to deploy cookies as detailed in our Privacy Policy.
Learn Everything About Recommendation Engines Inside Out
Have you ever felt like Amazon knows exactly what you want and wondered why? Do you think you have a product you know your customers will like but having hard time with getting discovered? If that is the case, you need to get familiar with the star of the e-commerce Recommendation Engines. Recommendation engines are basically counter guys at your online store. They tell what you might be interested in by checking what you are looking for.
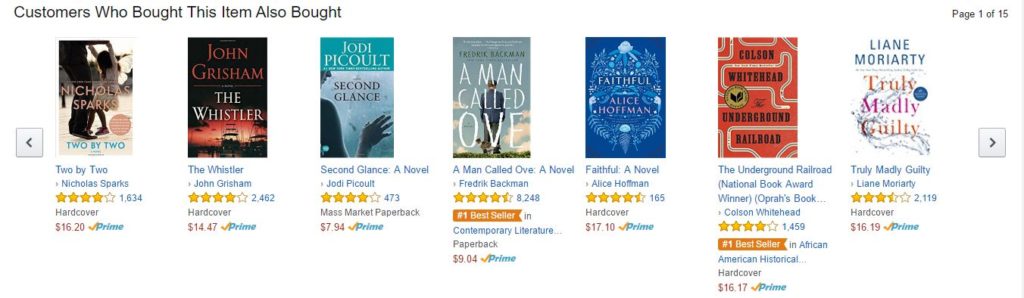
Technically speaking they are built-in algorithms that make purchase recommendations based on previous behavior. They are success-proven. Amazon has 25-30 percent of their sales channeled from recommendation engines.
Do not think of online shopping sites as the lone users. News sites and media streamers like Netflix also benefit from recommendation engines.
In this post, you will learn about
- types of recommendation engines
- some basic sales strategies that feed from recommendation engines
- crucial tips on how to use it
Types of recommendation engines
Before we dive deep into types of recommendation engines, let’s think of what can be done instead. Most frequently used practice is recommending the most popular item. Popular being the most liked/purchased by other customers. This fast and dirty technique has one large drawback. It does not include personalization.
Every customer is recommended the same products regardless of what they are looking for. Think of an online shop that suggest every customer to buy the new treadmill. This model can work in some areas tough. Think of a news site like CNN. There you will see a column saying Top News or Today’s Popular. These columns will appear same to every visitor. This works because most of the newsreaders are looking for that day’s hot news that are talked about the most.
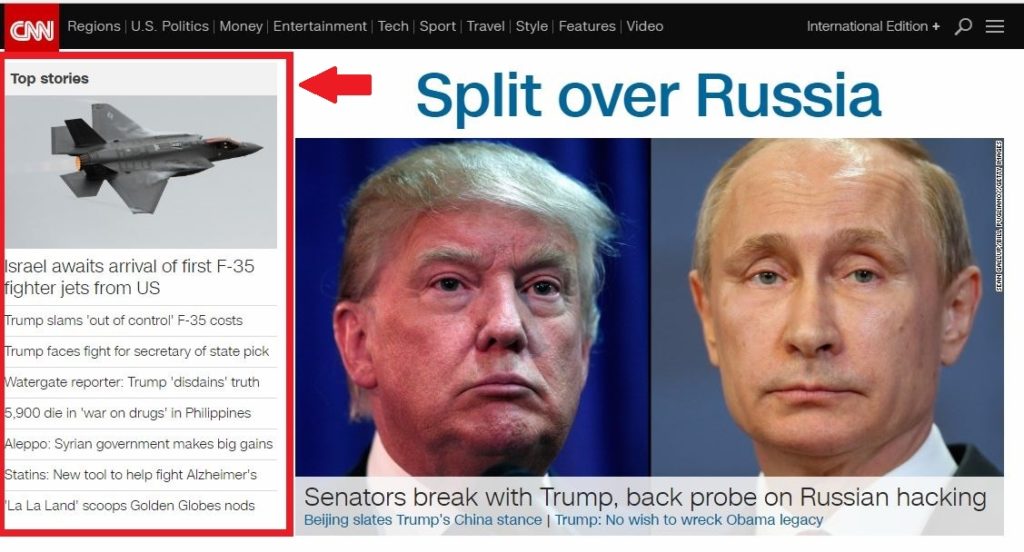
Here are the types of recommendation engines that provide personalization and are smart about the recommendations:
- Content-based algorithms
- Collaborative filtering algorithms
Content-based Algorithms
Idea: If you like A, you will probably like things similar to A, like ₳, Ᾱ, Ᾰ, ᾊ, or maybe even Ẫ.
- Works on the basis of similarity between items.
- Is easy to apply when we already have features of items well placed. An obvious example would be IMDb. There you will be suggested with movies within same category or having the same director.
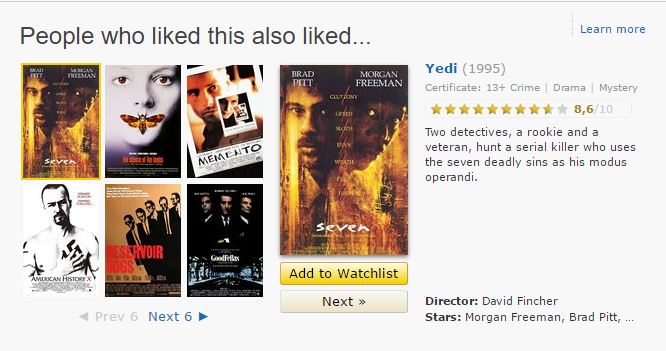
All are crime movies!
Collaborative Filtering Algorithms
Idea: If Alan likes 1,2,3 and Beatrice thinks 1,2,4 are interesting, the algorithm concludes that Alan and Beatrice share same interests and Alan should check item 4 where Beatrice would be looking at 3.
- This technique takes the past behavior into account instead of item features. Thus, this is the vastly used technique. Requires no pre-set data only customer behavior.
- We may split collaborative filtering techniques into 3 buckets
1-User-user Collaborative Filtering
User–user collaborative filtering, also known as k-NN collaborative filtering, was the first of the automated CF methods. It was first introduced in the GroupLens Usenet. User–user CF finds other users whose past behavior is similar to that of the current user and use their ratings on other items to predict what the current user will like.
To predict Alan’s preference for an item he has not rated, user–user CF looks for other users who have high agreement with Mary on the items they have both rated. These users’ ratings for the item in question are then weighted by their level of agreement with Mary’s ratings to predict Mary’s preference.
2-Item-item Collaborative Filtering
Rather than using similarities between users’ rating behavior to predict preferences, item– item CF uses similarities between the rating patterns of items. If two items tend to have the same users like and dislike them, then they are similar and users are expected to have similar preferences for similar items.
In its overall structure, therefore, this method is similar to earlier content-based approaches to recommendation and personalization, but item similarity is deduced from user preference patterns rather than extracted from item data.
3-Other techniques
There are other techniques that are written for a niche purpose in specifically for a seller, which prioritizes their needs. For a more scientific research you can check here.
Complementary Sales Strategies: Assume you have installed the recommendation engine and the system is doing fine. What remains is an excellent marketing strategy. Here I want to share a few strategies that I think will help you:
- If a customer buys A, show them A’s complementary products on all relevant pages.
- If a customer buys A, e-mail the product’s complementary products.
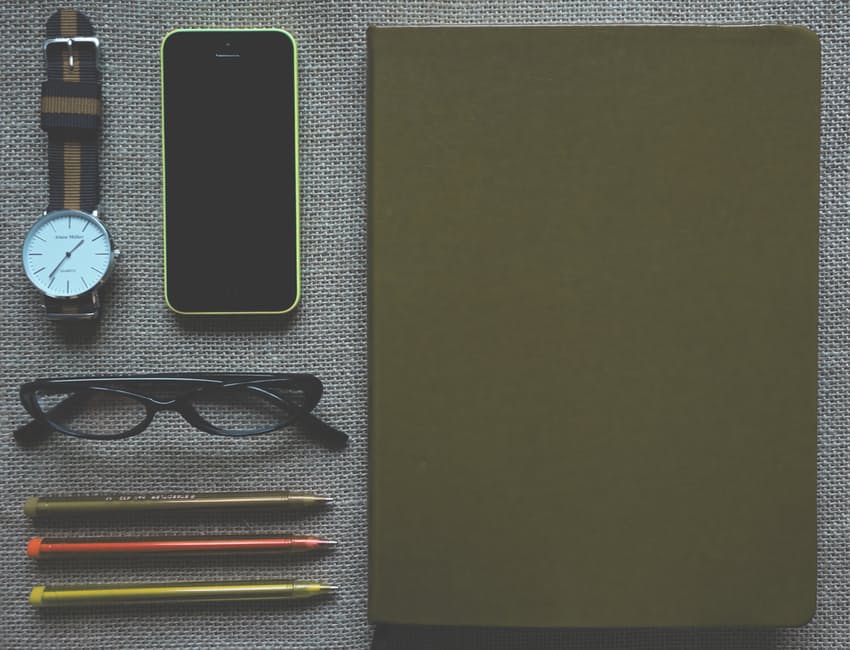
- For those who have reviewed any product in category A, send an email about the top selling products in the same category.
- After ten days from someone who has bought product B, send a mail about B’s new accessories
- After learning the user’s interest, make the necessary changes to the design to enhance the user experience.
Remarks on Recommendation Engines
So how does recommendation engines effect products with different characteristics? Different price, description, rating? Make your guess on them. Recommendation engines may not work as you might always expect.
First surprise for you would be that “who bought this also bought this” algorithm doesn’t help unknown goods to be discovered. You may say it worked for you in that way. However, in the aggregate level the product recommendations are usually the same. The system works in a way that “rich kids get richer”.
In order overcome this problem, algorithms should be arranged in the favor of niche items. Unless these adjustments are realized, there is always the possibility of the novel good getting lost like a needle in the hay.
Recommendation engine algorithms develop similar result to your guess with price and product description. Products with lower prices yield better outcomes than their high-priced competitors. Products with better description acts similarly. As you may have guessed if a product has a well-prepared description it gets more with the recommendation engines as well.
Another interesting fact is about characteristics of the products. Let’s classify products into 2 categories.
First being utilization goods. Goods that helps us with a solid need. Like a toaster or a notebook.
Second being goods like jewelry or a rare collection goods. We call them hedonistic goods. You don’t really need them. However, you crawl to have one of them.
Many researches suggest that hedonistic good make the most of recommendation engines. It’s hard to spend thousands on a shiny rock without having someone to pad your back. And now comes the joker in the pack! The biggest surprise about the recommendation engines is their performance with low-rated products. Products with low ratings always have high increases with the use recommendation engines where products with high ratings only see low-to-medium raises. Recommendation engines works as a substitute of the rating. Customers regard a products rating much less if the product is recommended to them.
Lessons to learn
Every counterpart should understand how the recommendation engines work and act accordingly. Producers should consider how to be discovered by new customers but also how to be discovered by algorithms. Consumers should be aware not to skip the novel that may be out of their radar. And most importantly, sales platforms should choose their recommendation engine wisely. There is no fit-to-all algorithm. A platform with wide assortment should select a different RE than a site selling very limited number of products. Now you know about the world of recommendation engines. Don’t stop spread the word and know the rules.