Our websites use cookies. By continuing, we assume your permission to deploy cookies as detailed in our Privacy Policy.
Recommendation Systems: Personalised Recommendation is Changing the Game
“Recommendation Systems” have become increasingly popular in recent years. The concept has been repeatedly utilised in various industries, specifically dominating eCommerce platforms. The majority of eCommerce sites such as eBay, Amazon, Alibaba make use of recommendation systems to give customers personalised service with the products they are more than likely to love. Given data collected that 35% of Amazon’s revenue comes from the recommendation engine, we can agree that recommendation systems are a technology worth looking into.
Let’s dive deeper.
What are Recommendation Systems?
The comprehensive web application class that involves predicting the user’s responses to offered options and product listings is called “Recommendation Systems”. A personalised recommendation is a critical facet of customer experience optimisation for digital merchandising. They are algorithms that aim to provide the most meaningful and accurate products for the user by filtering useful content from the pool with large amounts of data. On the other hand, the Personalised Recommendations Engine discovers the data patterns in the data set by learning consumers’ choices and producing results in line with the user’s needs and interests. These systems offer significant advantages in cross-sell marketing and upsell marketing.
What are Some Recommendation System Types?
Having looked into different personalised recommendation systems, one can distinguish 4 primary ones. These can be listed as:
- Collaborative Filtering Systems
- Content-Based Filtering Systems
- Popularity Based Recommendation Systems
- Hybrid Recommendation Systems
1 – Collaborative Filtering Systems
This filtering method usually relies on collecting and analysing information about the user’s behaviour, activities, or preferences and predicting what they will like based on their similarity with other users. One of its main advantages is that it does not need context for analysis. Therefore, it can accurately suggest complex elements without requiring the object to understand itself. Collaborative Filtering is based on the assumption that individuals who have had similar preferences in the past will make the same choices in the future. For example; If a user named Ben preferred products A, B, and C, and a user named Jenny preferred products B, C, and D, Ben would likely prefer product D, and Jenny would prefer product A.
Examples of Collaborative Filtering Algorithms:
- User to User Collaborative Filtering: Similar customers are associated, and products are presented based on the products chosen by the customers. It is quite effective but takes time and resources. This type of filtering requires analysing the information of both types of customers. Therefore, it is difficult to implement this algorithm for large platforms.
- Item to Item Collaborative Filtering: Very similar to the previous algorithm but focuses on product similarity rather than finding similarity among customers. With this algorithm, we can easily recommend similar items to a customer who buys any item. It requires fewer resources and time compared to user-user collaborative filtering. We can give an example of D&R’s system for this filtering type.
- Other simple algorithms: There are approaches such as “Market Basket Analysis” which generally do not have higher predictive power than the algorithms described above.
2 – Content-Based Filtering Systems
This filtering method is based on a product’s description and the content of user-preferred options. In a content-based recommendation system, keywords are used to describe the product. Algorithms try to recommend products similar to what a user has liked in the past. The idea of Contextual Filtering is based on the idea that if you like one product, you will like another similar product. The biggest issue with Content-Based Filtering is whether user preferences can be learned from products’ content sources.
3 – Popularity Based Recommendation Systems
As the name suggests, the popularity-based recommendation system works based on popularity. It uses trending existing products. For example, any product that each new user frequently purchases can be recommended to a newly registered user. There are some issues with popularity-based recommendation systems. The biggest problem with the popularity-based recommendation system is that data cannot be used for personalisation. Even if you know the user’s behaviour, you have no way of recommending products based on these options.
4 – Hybrid Recommendation Systems
Recent research shows that combining collaborative and contextual recommendations can be more effective. Hybrid approaches can be implemented by building the two recommendation systems separately and then combining them. In addition, these approaches can be combined into a single model by adding content-based features to a collaborative approach or by adding collaborative features to a content-based recommendation system. Several studies have focused on comparing the performance of the hybrid recommendation system with pure collaborative and content-based methods, and it has been proven that hybrid methods can provide more accurate recommendations than pure approaches. The hybrid recommendation system can be used to overcome common problems such as data shortages.
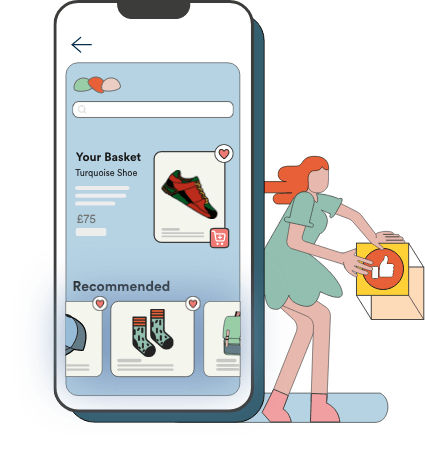
As previously mentioned, 35% of Amazon’s income comes from the recommendation engine. Amazon uses these suggestions both in email campaigns, push campaigns and in the content of its website. Since existing recommendation algorithms cannot scale to Amazon’s product portfolio, they developed their algorithms, and there are still in-depth discussions about how this recommendation engine works. Amazon uses item-by-item collaborative filtering that generates high-quality recommendations.
In the first quarter of 2019, Netflix reached 148.86 million users, according to Statista. This is a company that manages and uses data and places users in the centre when making decisions in this context. They make predictions by considering many categories such as the viewing history, the scores given to the content, users with similar tastes, players/genres, and aims to provide a better experience to its customers. In a way, we can say what makes Netflix successful is its ability to offer suggestions to users using machine learning algorithms by categorising a lot of data.
As with eCommerce and other areas, the music industry has moved towards creating a personalised experience for every listener. When one thinks about the music industry, the forerunner and the obvious example that comes to mind is Spotify. Spotify creates a personalised experience for more than 100 million users with its “Discover Weekly” playlists and recommends songs that you probably haven’t heard before but are highly likely to like. They use three recommendation models for these lists:
- Collaborative Filtering analyses and compares your preferences with those of other users.
- Natural Language Processing analyses the text in each song.
- Sound Modeling uses the raw sound of a song to understand the song’s melody and compares it to other songs.
How Segmentify Takes on Personalised Product Recommendations
According to Gartner’s research, B2B companies with effective personalisation on their eCommerce sites will outsell competitors who don’t have the same level of personalisation by 30%. 31% of online customers would like a more personalised shopping experience.
Segmentify approaches personalisation as a necessary facet to boost online sales. Understanding your visitors and making personalised product recommendations will create a unique shopping experience that will impress potential customers. In this sense, Segmentify analyses each visitor’s interests and helps you offer the most relevant products that your visitors will love. Personalisation means; the right offer, product, or campaign, to the right person, at the right time, and through the right channel. We will collect visitor data, segment visitors, analyse products and campaigns, personalise, and increase sales and conversion rates for these campaigns.
Here is a sneak peek of Segmentify’s up-and-coming personalised recommendation algorithms:
- Smart offers:
- Recommends the most viewed products to first-time visitors
- Recommends the most viewed products in their favourite categories to returning customers
- Purchased together:
- Recommends other products that were purchased with the item the customer is either browsing or has added to their basket
- Alternative products:
- Recommends products that have been viewed together and similar products to the campaign
Other recommendation campaigns we can offer you include campaigns for the home page, product page, basket page, checkout pages, journey builders, and category pages. Our technology offers you cutting-edge machine learning, custom recommendation rules, easy-to-use web application, multiplatform flexibility, scalable, high-performance engine, and real-time operations and more.
That is what Segmentify’s personalised recommendation systems can allow you to deliver. For more information, book a demo now.